IoT constantly faces new threats and needs flexible protection. reinforcement learning (RL) relies on consistent adaptation, so it’s the ideal choice. Here’s what an RL algorithm is and why it benefits IoT security.
What Is reinforcement learning?
RL is a machine learning technique. While most of its counterparts learn using strict data sets, it begins training with no knowledge base. Instead, it interacts with the environment and gains experience with each action. When it’s in action, it makes decisions automatically and constantly works to improve itself.
Typically, researchers guide their model or outline precise actions for it to take. After all, they want it to come to a specific conclusion. RL is different because it reaches its goal without human instruction. It needs no examples, values or input. The learning process only consists of an agent – the learner – its actions, an environment and an observable reward.
The agent experiments independently with a method similar to the trial-and-error process. It receives an incentive, and its environment changes when it does something favourable, reinforcing positive behaviour. If it fails, it adjusts its approach and tries again. It wants to maximise its reward, so it consistently reevaluates until it makes progress.
How is reinforcement learning used in IoT security?
Devices with constant connectivity are vulnerable to cyberattacks and need a real-time, adaptable solution. For this reason, many people have looked to RL to enhance IoT security. In fact, around 22% of companies were already using it in 2019.
RL algorithms can protect devices from eavesdropping, virus injection and jamming. People can deploy them in every place a standard AI would be beneficial. For example, they can respond to a distributed denial-of-service attack by throttling incoming traffic before it overwhelms the target servers. Their real-world applications are broad since their approach is somewhat challenging to replicate.
Many models used for the same task may find different strategies during their learning process. However, they will still accomplish the same overall goal because reward optimisation drives their constant adaptation. If the traffic-throttling algorithm suddenly finds its approach unsuccessful, it will adjust until it finds the solution.
Why is reinforcement learning used in IoT security?
Even though RL models can have a lengthy computational time because their learning style is so involved, the result is often worth it. They’re highly dynamic and can respond to various situations. IoT security constantly changes as technology advances, so an algorithm capable of evolving with it is vital.
Also, RL algorithms generally provide more significant improvements than other machine learning models. They increase malware detection accuracy by 40% in IoT security, while others have a static success percentage. Mainly, it’s because their realistic and dynamic strategies offer unique solutions.
One of the biggest reasons people use RL for IoT security is its ability to progress without support. The agent needs no existing knowledge about its environment to learn. This feature is ideal since finding realistic, relevant and accurate training data can be challenging. Researchers can use these models when they don’t know what to train on to reach their goal.
The value of reinforcement learning
IoT security needs an innovative tool like RL. While other model variants can adapt to new input, its learning style and constant reevaluation make it unique. It can begin training and carry out its mission without previous knowledge or human help. It’s a valuable technique in the shifting technological landscape.
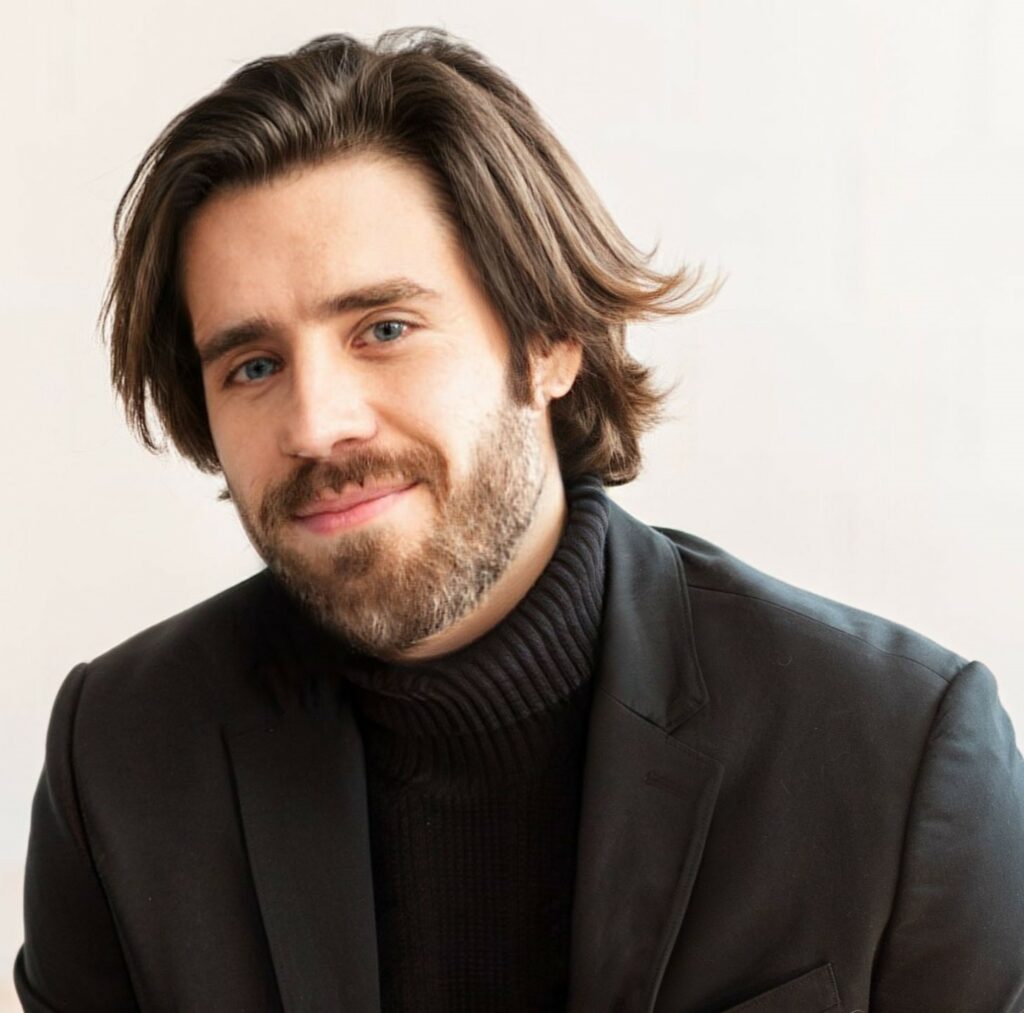
Zac Amos is the Features Editor at ReHack. With over 4 years of writing in the technology industry, his expertise includes cybersecurity, automation, and connected devices. For more of his work, follow him on LinkedIn.