Nebu Philips, Senior Director, Strategy and Business Development, Synaptics writes about the advantages of moving AI workloads to the Edge
The fundamental difference between the core and the Edge is the availability of resources. At the core, compute capabilities, power, memory, and space are abundant, while at the Edge, they are scarce. However, there is omnipresent pressure to shift artificial intelligence (AI) workloads from the core of the network to the Edge. That trend is now well underway, but it will require innovation for Edge AI computing to meet the growing demand for more powerful local AI. It is going to require AI-native embedded computing.
Shifting AI workloads from the core to the Edge has tremendous advantages for data centres, Edge applications, and users alike. Work that would have been done in data centres is instead performed at the Edge, easing demand pressure on data centres. The energy it would have taken to transmit data to and from data centres gets saved. Processing at the Edge by necessity must be performed using minimal resources, potentially saving even more energy.
Edge processing eliminates the latency incurred in data’s round-trip to and from a data centre; minimising latency is almost always a factor in positive customer experience, but it is critical for applications that operate in real time or close to it. Any personal user data collected at the Edge remains at the edge, making it easier to protect users’ privacy.
The demand for generative AI is fuelling explosive growth in the data centre power requirements. The world has 55 gigawatts of data centre capacity today, but will need around 122 GW of data centre capacity online by the end of 2030, according to Goldman Sachs. Expressed in terms of sites (data centres and co-location facilities), ABI Research expects there will be 6,111 public data centres in operation worldwide by the end of 2025, and projects a need for 8,378 data centres by 2030.
Most analysts expect the Edge AI market to similarly double in size (at least) in that same time frame. To offer one example, Markets and Markets says that the Edge AI hardware market (largely CPUs, GPUs, TPUs, and FPGAs), worth US $24.2 billion in 2024, will be worth $54.7 billion by 2029.
Satisfying the projected demand for additional data centre capacity is already a daunting prospect, but the situation would be significantly more challenging if data centres had to take on all of the AI workloads that are expected to be processed at the Edge as the Internet of Things (IoT) rapidly expands.
While offloading AI processing to the IoT Edge reduces some of the burden on cloud computing data centres, there are benefits for Edge applications, including lower latency and power consumption, user privacy, greater security, and optimal user of available network bandwidth. Privacy is particularly important. Personal data is easier to protect when it is collected, processed, and stored locally. Various regulatory agencies around the world have adopted regulations mandating this.
Technological hurdles
In the Cloud, it is possible to employ as many GPUs as needed to manage AI processing. Presently there is one dominant GPU supplier, and the maturity of the language and methodologies used with its GPUs makes data centre AI a relatively stable ecosystem despite rapid evolution.
Moving from core to Edge, the landscape changes; it becomes increasingly heterogeneous. Operating at the Edge is a complex and disjointed experience for developers as they work to put together the ICs, software, tools, and partners they need to get AI-enabled IoT designs to market.
Data centre operators can feed power-hungry workloads, such as training and running large language models (LLMs), with as many resources as needed – more processing power, more electricity, more physical space, and more cooling.
These resources are strictly constrained at the Edge. IoT devices must make do with limited compute capability, power, memory, and space. Trying to perform AI and machine learning (ML) at the Edge within those same constraints is an additional challenge.
Edge applications
Many IoT applications are sensor-based. Vision-based examples include presence detection, attention detection, body-pose estimation, and gesture recognition. Other Edge applications rely on microphones to detect voice input or process environmental sounds. Many of these AI workloads are performed in the Cloud today, but from a resource allocation standpoint, it makes much more sense to perform them at the Edge.
While the most commonly available silicon options can be workable, most either lack sufficient computing power or vastly exceed what is needed – and few are designed from the ground up to perform ultra-low power AI inference. The need for a readily available solution that balances the unique performance and energy efficiency requirements for IoT applications is clear. Fortunately, innovative alternatives are available.
Improving Edge AI
The challenge, then, is to provide sufficient processing capabilities for AI/ML at the Edge, while minimising power consumption. The technology has to be easy to use, and it should avoid exacerbating the heterogeneity of solutions at the Edge – it should be open source.
Synaptics has responded to these challenges by introducing its Astra AI-Native embedded compute platform for the IoT. The Astra platform unifies the fragmented IoT ecosystem by providing a standardised and cohesive developer experience across various market segments, including consumer, enterprise, and industrial devices. It is based on scalable embedded systems on chips (SoCs) with open-source software and development tools, supported by Synaptics Veros connectivity solutions and a dynamic ecosystem of partners.
The silicon has neural processing units (NPUs), CPUs, GPUs, and DSPs built in, and it scales from tens of GOPS to 8 TOPS, filling a range where there are currently few, if any, options. These solutions are optimised for vision-based systems, but Synaptics also supports other modalities, such as voice and audio.
The company’s AI Developer Zone is a resource-rich environment designed to empower developers to create AI applications. The Zone offers open-source SDKs and Cloud-based tools that simplify the development process, making it more accessible to a broader range of developers.
Based on its experience with set-top box (STB) manufacturers with stringent content security requirements for digital rights management (DRM), Synaptics has created a secure pipeline for AI inferencing. The platform’s SoCs feature AI engines running on ultra-low power AI MCUs to high-performance MPUs, providing a unified architecture that can scale across various performance levels.
Edge AI computing is set to redefine the IoT, offering a way to manage vast volumes of data while enhancing user experiences. With its Astra platform and focus on open-source tooling and developer support, Synaptics is helping to drive the transition to more intuitive user experiences at scale.
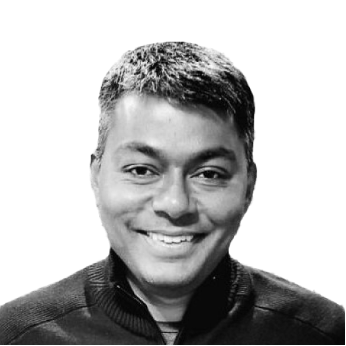
Augustine Nebu Philips is Senior Director of Strategy and Business Development at Synaptics, where he is responsible for overseeing strategic initiatives and driving business growth in IoT and Edge processor solutions
There’s plenty of other editorial on our sister site, Electronic Specifier! Or you can always join in the conversation by commenting below or visiting our LinkedIn page.